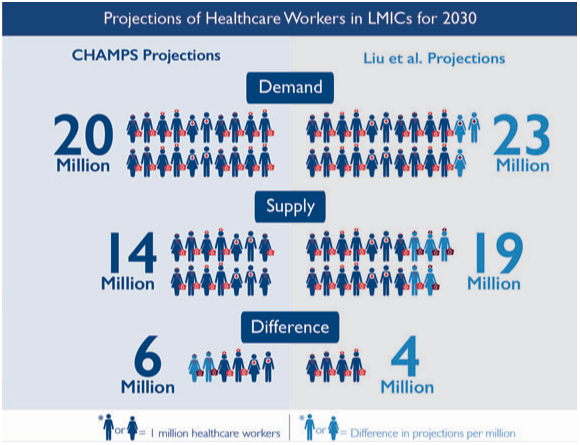
03 Feb Three Questions with Dr. Pierre Nguimkeu: Forecasting the Supply & Demand for HRH
Dr. Pierre Nguimkeu is an Associate Professor of Economics at Georgia State University in the United States. An expert in econometrics, development economics, and data science, his research has contributed to the development of new econometric and data analytic techniques in the presence of poor-quality data (e.g., small samples, measurement errors, and missing data). He has also worked in developing public policies aimed at promoting employment, entrepreneurship, productivity, and growth in the informal and formal sectors of developing countries. Dr. Nguimkeu and ThinkWell, an HRH2030 partner, developed and tested a new econometric model that measures the impact of economic, epidemiological, and demographic transitions on the health labor market, represented by physicians, nurse-midwives and community health workers. The Comprehensive HRH Assessment, Modeling, and Planning Solution (CHAMPS) provides government decision-makers and planners with the information they need to plan for the health workforce of the future.
Question 1: Predicting the future supply of and demand for health care workers is critical for countries so that policymakers can invest wisely in human resources for health (HRH) and maximize limited resources. While HRH planning models had become increasingly sophisticated over the past 20 years, they still had some limitations. What specifically were these limitations and why was there a need to create a new kind of model?
First of all, it is important to recognize the merit of earlier HRH planning models. They were designed cleverly and elegantly to solve a critical need for evidence-based policy-making in the health labor market, and they set the stage for the development of more sophisticated models. These earlier models mostly focused on economic factors which are obviously the first factors to look at when we think of the health labor market, and they were based on supply and need, often ignoring demand.
More recently, though, there was a need to look beyond economic indicators, and examine other relevant factors such as epidemiological and demographic transitions and their impact on the demand and supply of healthcare workers. It was also important to understand the role of technological advances as well as changes in the organization of healthcare delivery, all of which are likely to affect skills mix and the dynamics of the health labor market. It is from these perspectives that a new and more comprehensive model was created. The ensuing methodology, CHAMPS, provides more realistic estimates of the demand and supply of healthcare workers, because it includes vastly more information than the earlier methods, is more flexible and accurate in fitting historical data, and makes fewer simplifying assumptions, which reduce the risk of overestimation.
In addition, unlike earlier models that mainly focused on physicians and sometimes nurses/midwives, CHAMPS has an unprecedented ability to also directly project the demand and supply of community health workers (CHWs), who are key to universal health coverage as they provide primary healthcare to a large number of underserved and hard to reach populations. Finally, our approach also differs from earlier HRH projection methods which were designed to generate country or sub-regional projections only from a single global model estimation (top-down), whereas CHAMPS can directly provide projections at a country level (e.g., Ghana), or an income-group level (e.g., low- or middle-income countries (LMICs) that are more homogeneous and therefore less influenced by outlying observations (bottom-up).
Question 2: During your research, you used CHAMPS to project the global demand for and supply of healthcare workers in 2020 and 2030. What did your research find, and how did these projections differ between CHAMPs and earlier models?
We used the CHAMPS to project the global demand for and supply of healthcare workers in LMICs for the next 15 years. We found that, in addition to economic factors, epidemiological, and demographic transitions turned out to have significantly large impacts on the demand for healthcare workers in these countries, and considerably improved the predictive capability of the model without compromising efficiency. The model predicted that by 2030 the demand for physicians and nurses/midwives in LMICs will be 20.3 million, while the supply will only be 14.3 million, thereby projecting a general shortage of almost 6 million physicians and nurse/midwives. The shortage in CHWs, on the other hand, is predicted to be almost 2 million by 2030 in LMICs. This latter projection incidentally matches the number advocated independently by the One Million Community Health Worker Campaign and UNAIDS for 2020. It should be noted that our CHAMPS projections are an ‘average’ from a wide but reasonable range of possibilities that we calculated.
When we compare the CHAMPS projections for 84 LMICs with earlier models’ projections for 73 LMICs, the difference between the two estimates are clearly noticeable and not just because of the sample size difference. The CHAMPS prediction of the demand for healthcare workers is lower by 3 million than the earlier models predictions, which is more realistic, because CHAMPS considers the influence of a much larger number of factors together. CHAMPS also predicts a substantially lower supply than earlier models’ projections, almost 6 million fewer healthcare workers, because it uses a more conservative approach (e.g., linear and/or S-shape trends rather than linear trends only) that is less prone to an overestimation of healthcare worker supply. As a result, CHAMPS forecasts a more severe healthcare worker shortage by 2030 than earlier models’ projections (5.9 million vs. 3.7 million).
Question 3: Moving from the global perspective to a country focus, when applied at the country level, the CHAMPS projections can help HRH policymakers and planners better understand the composition, skills mix, and major gaps of the future health workforce. As an example, tell us what the CHAMPS’ projections found in Ghana.
Ghana is an interesting case because it is one of the few countries in the sub-region that has successfully implemented a universal health insurance program (in 2005) through a National Health Insurance Scheme (NHIS). The CHAMPS model is flexible enough to account for these types of country specificities as well as other contextual factors such as the incidence of brain drain among health professionals, the influence of traditional medicine, religious beliefs, and social norms (although these latter factors could not be actually tested in Ghana due to data limitations).
In the case of Ghana, however, the CHAMPS incorporated the impact of NHIS in its projections of healthcare workers. We found that by 2030, Ghana will have a shortage of approximately 60,000 nurse/midwives compared to the projected need and will be about 70,000 CHWs short of the projected need. Nonetheless, the CHAMPS projections also show that the country is likely to have enough supply of physicians by 2030, possibly experiencing a slight and insignificant shortfall of 9,000 physicians (out of a demand for 166,000). This presents good news for HRH stakeholders in the country, given that supply of physicians should mostly keep up with demand while predicted shortages for nurse/midwives and CHWs could entirely be overcome given the country’s resources. These results suggest that the NHIS will continue to play a role in stimulating the demand for healthcare workers in Ghana (as confirmed by more recent research), although in relative terms the shortage in Ghana by 2030 will be lower than the comparable predicted global shortage in LMICs (5% vs 43%).
Overall, the shortage in healthcare workers for Ghana projected by the CHAMPS methodology is significantly lower than projections from earlier models. Such estimates allow the government to make more targeted policy decisions in response to predicted changes in the country, such as accelerating economic growth, a population that becomes more prone to chronic diseases and aging, or the continuing take-up of universal health coverage.
This example from Ghana can be replicated for any number of countries with similar data availability. Data are readily available from the dataset of 84 LMICs that was used to develop the CHAMPS methodology; and is added to by international organizations regularly. Policymakers can now access specific information about the most influential economic, epidemiologic, and demographic factors that will drive HRH demand over the coming decade in their countries.
Associated Content